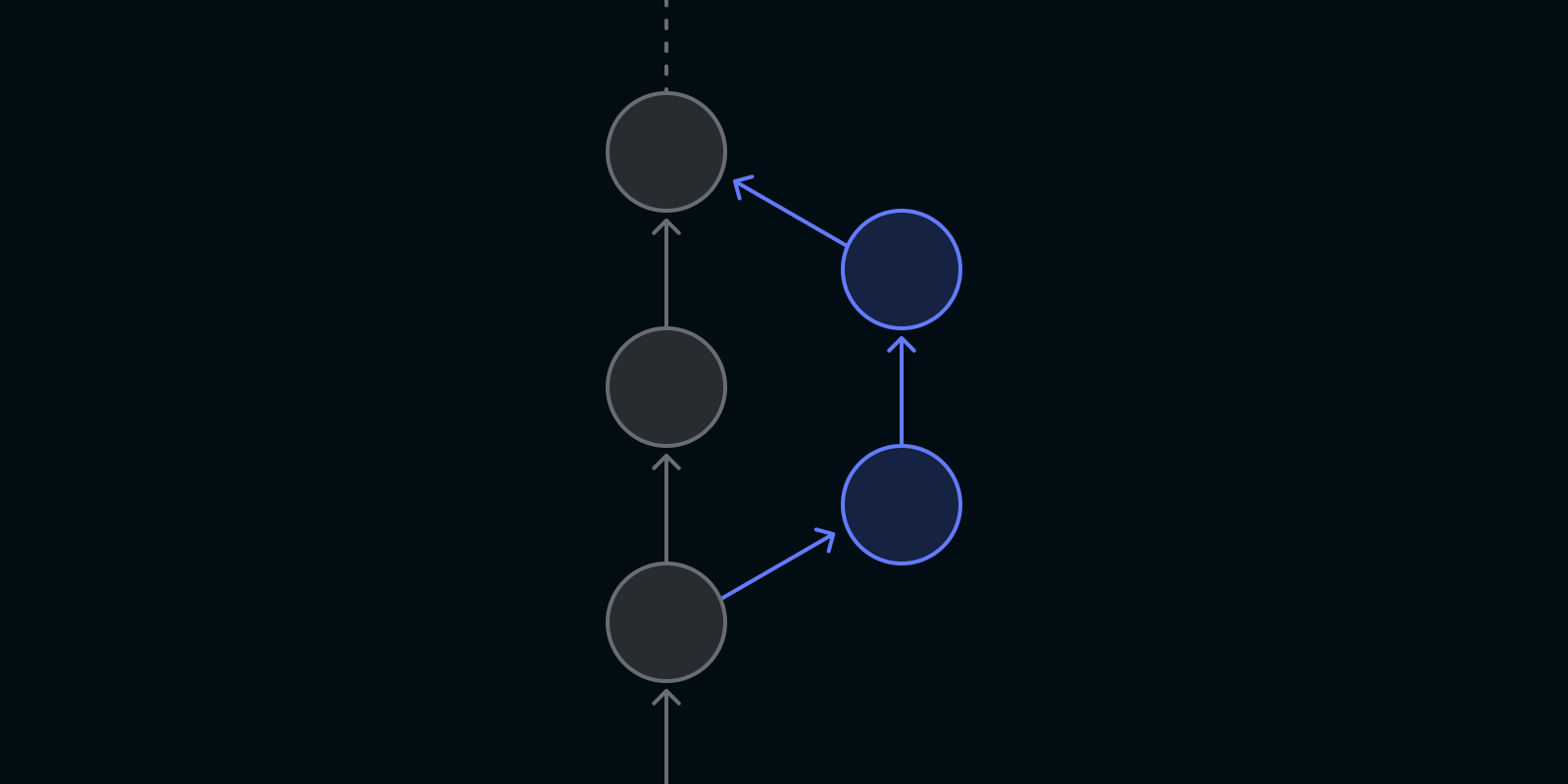
Announcing stack-pr: an open source tool for managing stacked PRs on GitHub
We are pleased to announce the release of a new tool aimed at simplifying the management of stacked pull requests (PRs) on GitHub - stack-pr. This tool is still in its early development days, but we are excited to share it with the community and welcome your contributions.
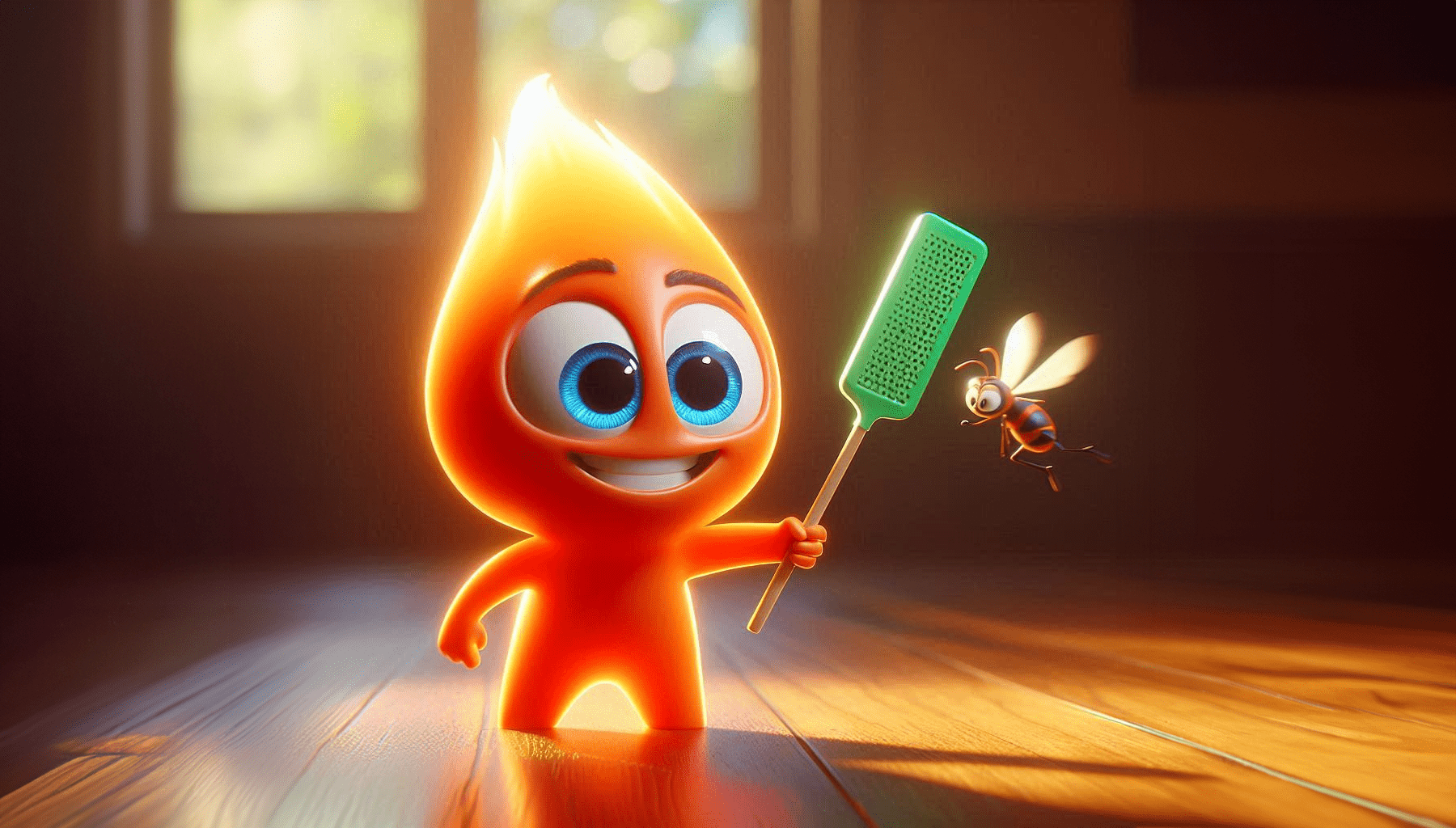
Debugging in Mojo🔥
Developer tooling is a big priority for Mojo and MAX, we want to vastly improve the debugging experience compared to the traditional Python, C++, and CUDA stack. Machine learning often requires inspecting the state of a program after a long running process, requiring more control than what "print debugging" gives you. Over time this tooling will extend to GPUs, allowing you to step through CPU code into GPU calls with the same developer experience.
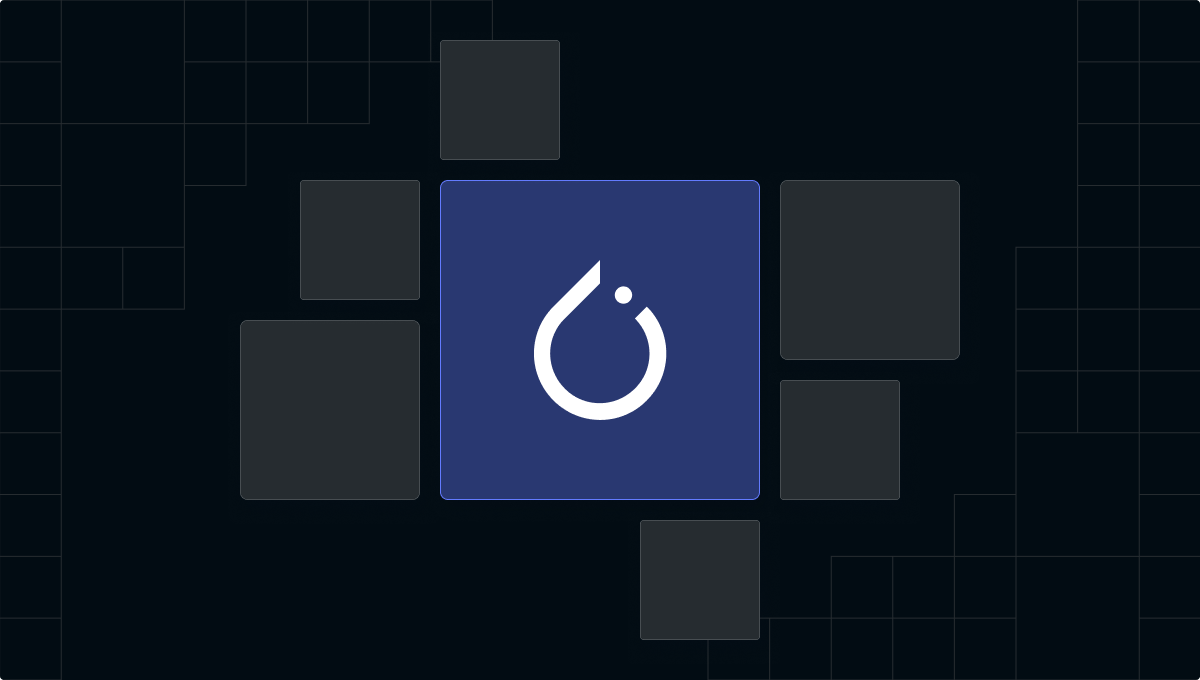
Bring your own PyTorch model
The adoption of AI by enterprises has surged significantly over the last couple years, particularly with the advent of Generative AI (GenAI) and Large Language Models (LLMs). Most enterprises start by prototyping and building proof-of-concept products (POCs), using all-in-one API endpoints provided by big tech companies like OpenAI and Google, among others. However, as these companies transition to full-scale production, many are looking for ways to control their AI infrastructure. This requires the ability to effectively manage and deploy PyTorch.
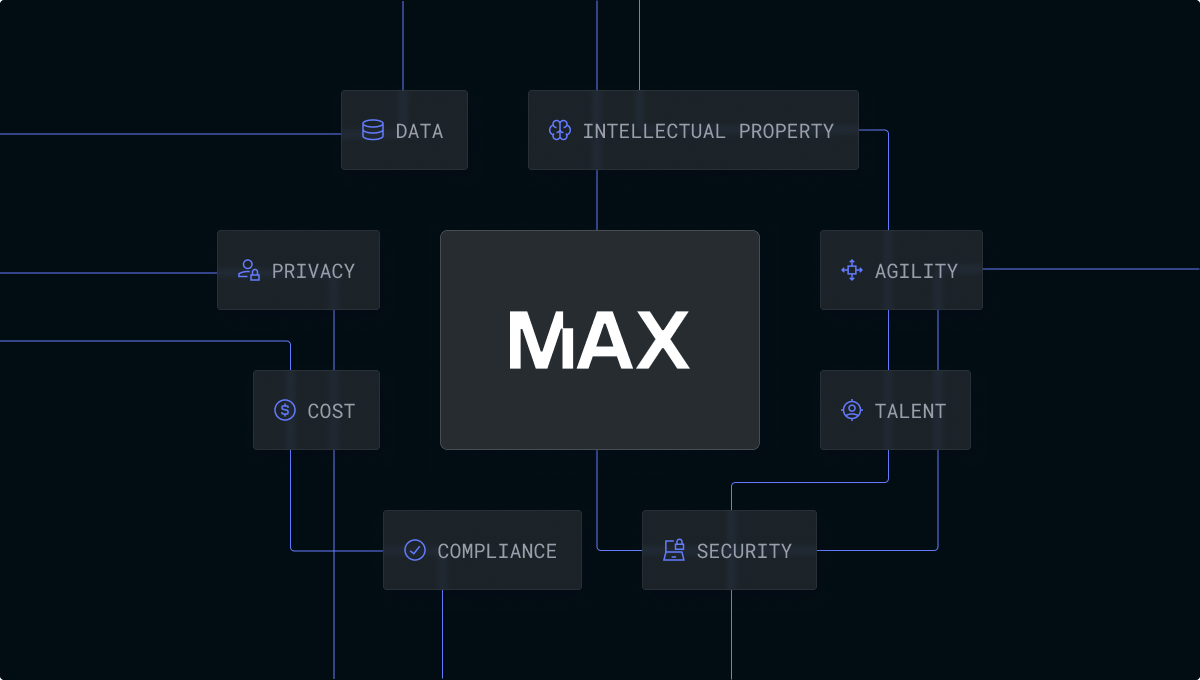
Take control of your AI
In today’s rapidly evolving technology landscape, adopting and rolling out AI to enhance your enterprise is critical to improving your organization’s productivity and ensuring that you are delivering a world-class product and service experience to your customers. AI is without question, the single most important technological revolution of our time—representing a new technology super-cycle that your enterprise cannot be left behind on.

Develop locally, deploy globally
The recent surge in AI application development can be attributed to several factors: (1) advancements in machine learning algorithms that unlock previously intractable use cases, (2) the exponential growth in computational power enabling the training of ever-more complex models, and (3) the ubiquitous availability of vast datasets required to fuel these algorithms. However, as AI projects become increasingly pervasive, effective development paradigms, like those commonly found in traditional software development, remain elusive.